Predicting Customer Attrition in Banking
Introduction In the dynamic landscape of modern banking, predicting and preventing customer churn has become a critical priority for financial...
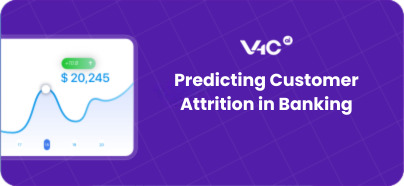
Introduction
In the dynamic landscape of modern banking, predicting and preventing customer churn has become a critical priority for financial institutions. By harnessing advanced data science techniques and leveraging platforms like Dataiku, banks can delve deep into diverse data sources. This enables them to gain valuable insights into customer behavior, proactively identify at-risk customers, and implement targeted retention strategies. These efforts not only aim to improve profitability through enhanced customer retention rates but also prioritize fostering enduring relationships with clients. This strategic approach underscores the importance of data-driven decision-making in maintaining competitiveness and sustainability in the banking industry.
Business Goal
The primary objective is to develop a robust predictive model to anticipate customer churn within the banking sector. Using Dataiku, banks can proactively utilize data analytics, machine learning, and demographic insights to identify and retain at-risk customers. The ultimate goal is to enhance customer retention rates, improve profitability, and foster long-term relationships.
Account Churn & Its Relevance
Account churn in banking refers to customers closing their accounts or reducing their usage of banking services. This can manifest in various ways, such as discontinuing automatic payments or switching to competitors for loans. In a market where consumers have many options, banks must differentiate themselves and provide exceptional experiences. Retaining customers is more cost-effective than acquiring new ones, making churn prediction critical.
Datapoints Used in Modeling
- Transaction Details: Data related to bank transactions, including transaction types and amounts.
- Account Details: Information on account activities, such as opening and closing dates, overdrafts, and return charges.
- Customer Demographics: Information about the customer’s age, gender, location, marital status, and occupation, which influence banking needs and churn behavior.
Challenges & Limitations
- Data Quality and Availability: Ensuring clean, accurate, and comprehensive data from various banking systems is essential for effective predictive models.
- Data Privacy and Security: Handling sensitive customer information requires adherence to regulations such as GDPR, adding complexity to data analysis.
- Imbalanced Data: Churners are often fewer than non-churners, potentially leading to biased models. Addressing this imbalance is crucial for accurate predictions.
- Temporal Dynamics: Customer behavior is influenced by external factors such as economic conditions and market trends. Capturing these dynamics in models is challenging but necessary for accurate predictions.
Churn Indicator
The account closure date in the account details serves as the churn indicator, a crucial metric and dependent variable that signifies the end of a customer’s relationship with the bank.
Dataiku: Powering End-to-End Data Science
Using Dataiku, the entire data science workflow for churn prediction is streamlined. This includes data storage, preprocessing, modeling, and deployment.
- Unified Data Platform: Dataiku integrates with various data sources, ensuring a cohesive data environment for analysis.
- In-Platform Processing: Dataiku’s visual interface allows for Exploratory Data Analysis (EDA), data preprocessing, feature engineering, and model training within the platform. This minimizes the need for complex pipelines or external systems.
- Simplified Deployment: Models can be deployed directly within Dataiku, facilitating seamless integration and operationalization.
- Enhanced Data Security: Dataiku provides robust security features, ensuring customer data remains encrypted and secure throughout the process.
Model Selection and Evaluation
Using Dataiku, we performed feature selection and model development, experimenting with various classification algorithms. Dataiku’s visual and AutoML capabilities streamline this process.
- Top Models: LightGBM, Random Forest, and AdaBoost were the top-performing models selected based on their accuracy and ability to handle imbalanced data.
- Ensemble Model: To avoid overfitting, a stacking ensemble approach was used, combining LightGBM, Random Forest, and AdaBoost. This provided robust and generalizable predictions.
Model Deployment
We integrated the churn prediction model into a user-friendly interface for real-world applications using Dataiku’s deployment capabilities.Deployment and User Interface
- Interactive Interface: Dataiku’s dashboarding capabilities created an intuitive interface where users can input account features and receive churn predictions.
- Explainability: Dataiku provides tools for both local and global explainability, helping users understand the factors influencing predictions.
Future Scope
- Integration of Additional Data Sources: Enhance models by incorporating data from external sources like social media, web browsing, and demographics for a comprehensive view of customer behavior.
- Near Real-Time Prediction: Implement real-time monitoring for timely interventions.: Implement real-time monitoring to identify churn indicators as they occur, enabling timely intervention strategies for retaining at-risk customers.
- Natural Language Processing (NLP): Extract insights from unstructured text data in customer interactions using NLP, enabling sentiment analysis for churn prediction and analysis.
Conclusion
Predicting customer churn is vital for maintaining a healthy customer base in banking. Dataiku enables a secure and efficient end-to-end data science workflow, from data storage to model deployment. By leveraging Dataiku’s comprehensive platform, banks can streamline their processes, enhance data security, and improve customer retention through effective churn prediction models.
More blog posts
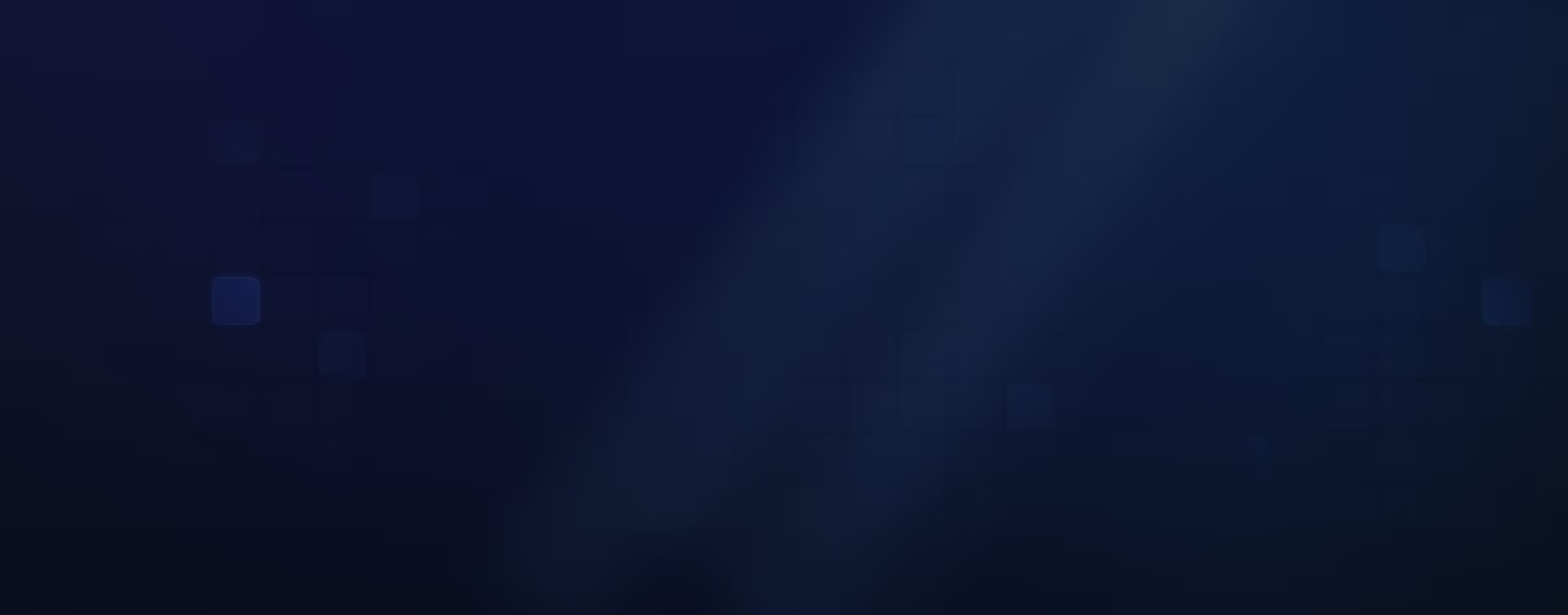