MLOps in Life Sciences: Ensuring Compliance & Driving Performance
Discover how effective Generative AI governance models can balance innovation with regulatory compliance.
.png)
In the life sciences industry, where innovation directly impacts human health and well-being, the ability to operationalize machine learning (ML) effectively can be the difference between breakthrough advancements and stalled progress. From streamlining drug discovery to improving patient diagnostics, ML models have become indispensable tools. However, deploying these models at scale, ensuring they function reliably, and meeting strict regulatory requirements present a unique set of challenges.
In this blog, we will delve into how MLOps in Life Sciences can address the unique challenges of the life sciences industry. Drawing from V4C’s expertise in operationalizing machine learning for high-compliance sectors, we will share actionable strategies to ensure regulatory compliance, enhance model explainability, and optimize performance monitoring at scale.
The Role of MLOps in Life Sciences
Life sciences organizations—ranging from healthcare providers to biotech firms, pharmaceutical companies, and medical device manufacturers—operate in a highly regulated environment with unique challenges. These include handling sensitive patient data, adhering to stringent compliance requirements like HIPAA and FDA regulations, and ensuring models perform reliably in real-world conditions. MLOps offers the necessary infrastructure to tackle these challenges by enabling:
- Regulatory Compliance: Ensuring data security, auditability, and adherence to privacy standards.
- Model Explainability: Enhancing transparency and building trust in AI-driven decisions.
- Scalability and Performance Monitoring: Managing large-scale and complex machine learning pipelines.
The adoption of MLOps in life sciences is not just a technical shift but a business imperative. The growing importance of MLOps is evident in its projected market expansion. Valued at USD 1.1 billion in 2022, the MLOps market is expected to grow to USD 5.9 billion by 2027, reflecting a robust compound annual growth rate (CAGR) of 41%. This rapid growth underscores the increasing demand for scalable and efficient ML operations, especially in industries like life sciences, where stakes are exceptionally high.

Challenges in Operationalizing Machine Learning in Life Sciences
The life sciences industry is uniquely positioned to benefit from AI and machine learning, yet it faces distinct challenges in operationalizing these technologies. From navigating stringent regulations to handling complex datasets, these hurdles require robust solutions to ensure AI workflows deliver value without compromising compliance or performance.
Regulatory Compliance
Life sciences organizations operate under strict regulatory oversight. Frameworks such as HIPAA (Health Insurance Portability and Accountability Act) and FDA (Food and Drug Administration) guidelines mandate stringent data privacy, security, and auditability standards. These regulations impact how machine learning models are developed, deployed, and monitored, making compliance a critical challenge. Non-compliance can lead to financial penalties, reputational damage, and, in some cases, risks to patient safety.
Explainability in AI Models
Unlike other industries, life sciences demand a high degree of transparency and explainability in machine learning models. Whether in medical diagnostics or drug discovery, AI predictions must be interpretable, with clear reasoning for outputs. This is essential not only to gain regulatory approval but also to ensure trust among researchers, clinicians, and patients. Black-box AI models are less acceptable in this field, where decisions directly impact human health and wellbeing.
Handling Large and Complex Data Sets
Life sciences data is massive, diverse, and often unstructured. Examples include:
- Genomic data, where a single human genome sequence can occupy up to 200 gigabytes.
- Clinical notes, which require natural language processing (NLP) for structured insights.
- Imaging data, such as X-rays or pathology slides, that demand computationally intensive processing through computer vision (CV) models.
Recommended Read: AI/ML Model Deployment Made Easy with Dataiku and v4c.ai
Solutions Enabled by MLOps in Life Sciences
MLOps frameworks are uniquely equipped to address these challenges by enabling compliance, explainability, and scalability in AI workflows.
Regulatory Compliance
MLOps facilitates regulatory adherence by:
- Maintaining audit trails: Detailed records of AI decisions ensure traceability, which is critical for FDA approval and other regulatory processes.
- Secure model deployment: Models are deployed within existing health data infrastructures, reducing vulnerabilities and ensuring compliance with HIPAA and similar regulations.
Model Explainability and Monitoring
To address the need for transparency, MLOps enables:
- Continuous monitoring: Early detection of model drift ensures AI remains reliable in changing real-world conditions.
- Feature effect analysis: Tools for understanding which data features impact specific predictions, critical for applications like AI-driven medical devices requiring regulatory validation.
Data Handling and Scalability
Efficiently managing life sciences data is a cornerstone of MLOps solutions:
- Unstructured data management: Tools like NLP pipelines and computer vision capabilities streamline insights from genomic sequences, clinical notes, and imaging data.
- Optimized computational performance: High-performance frameworks allow even complex models to run on standard CPUs, reducing the cost and dependency on specialized GPUs.
Use Cases: MLOps in Life Science Industry
The life sciences industry leverages MLOps to tackle unique challenges, such as ensuring compliance with strict regulatory frameworks, managing sensitive data, and optimizing the efficiency of machine learning (ML) workflows. Below are key MLOps use cases tailored to this sector:
- Drug Discovery and Development:
MLOps supports rapid iteration and deployment of ML models for drug discovery by integrating continuous testing and validation pipelines. By automating workflows, pharmaceutical companies can reduce the time and cost associated with identifying potential compounds while maintaining compliance with regulatory standards. - Clinical Trial Optimization:
Efficient patient selection, recruitment, and monitoring during clinical trials are critical. MLOps enables the development of predictive models that analyze vast datasets to identify suitable candidates, monitor trial outcomes in real time, and optimize trial design. - Personalized Medicine:
MLOps ensures robust data integration and governance for building ML models that recommend personalized treatment plans. By analyzing genetic, clinical, and lifestyle data, these models support targeted therapies, enhancing patient outcomes while adhering to data privacy laws. - Medical Imaging Analysis:
Automated image recognition and analysis powered by ML models can detect anomalies in medical imaging. MLOps ensures these models are continuously retrained and validated, improving diagnostic accuracy for conditions like cancer or cardiovascular diseases. - Pharmacovigilance:
By using MLOps to monitor adverse drug reactions in real time, life sciences organizations can maintain compliance while identifying and mitigating risks quickly. Automated pipelines analyze unstructured data sources, such as social media or patient reports, for early detection of safety concerns. - Regulatory Compliance and Documentation:
MLOps frameworks streamline model explainability, version control, and reporting, ensuring that AI/ML solutions comply with FDA, EMA, and other regulatory guidelines. Automated documentation processes further reduce operational overhead.
How V4C Supports MLOps in Life Sciences
At V4C, we specialize in implementing MLOps frameworks tailored for the unique demands of the life sciences industry. Our solutions integrate robust compliance frameworks with platforms like Dataiku, enabling seamless development, deployment, and monitoring of machine learning models. We provide scalable infrastructure for performance tracking and regulatory reporting while ensuring the secure handling of sensitive healthcare and genomic data. By leveraging our expertise, life sciences organizations can accelerate innovation, maintain compliance, and achieve operational excellence.
Conclusion
MLOps is revolutionizing the life sciences industry by ensuring compliance, optimizing machine learning workflows, and driving innovation across healthcare, pharmaceuticals, and medical devices. By adopting MLOps, organizations can enhance transparency, scale their operations, and maintain regulatory standards, ultimately improving patient outcomes and operational efficiency.
Partner with V4C for expert guidance in implementing MLOps solutions tailored to the life sciences sector. Contact us today to learn how we can help you navigate compliance, optimize performance, and accelerate innovation in your organization.
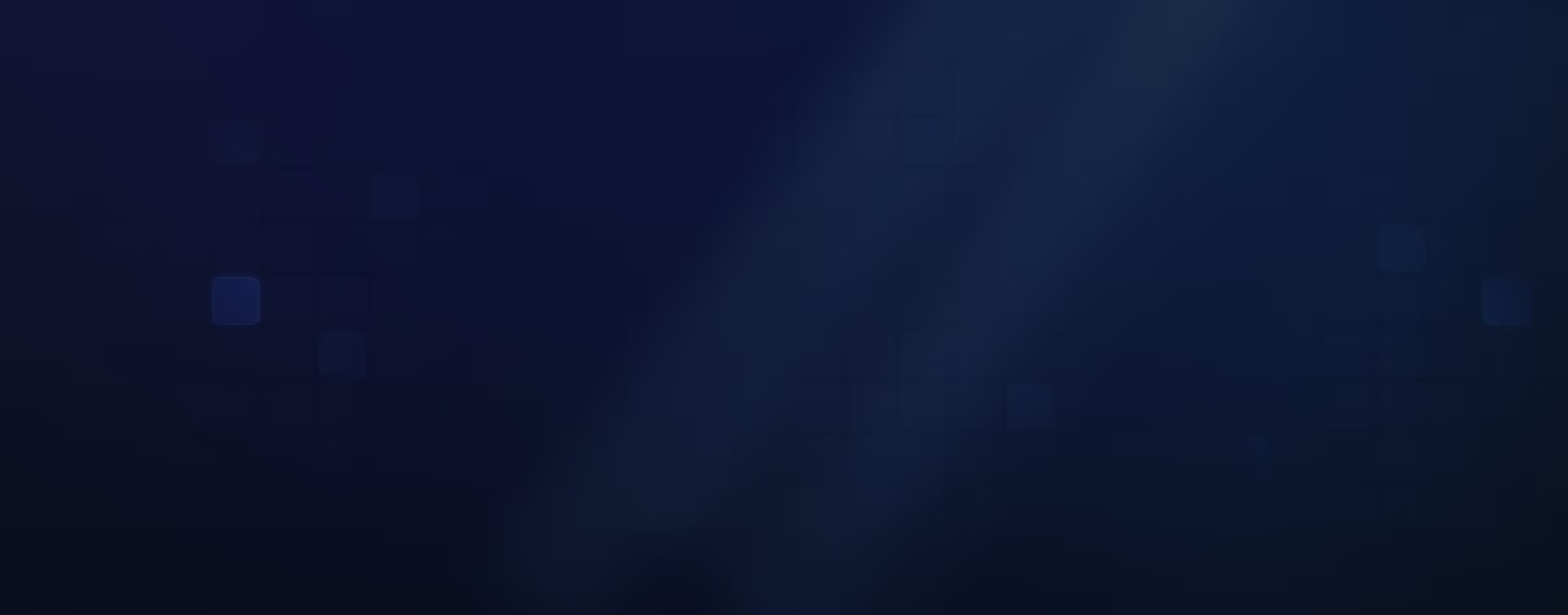