Generative AI Governance: Balancing Innovation & Regulation
Discover how effective Generative AI governance models can balance innovation with regulatory compliance.

As generative AI rapidly gains traction across industries, its transformative potential is matched by an urgent need for robust governance frameworks. Organizations are adopting generative AI technologies at an unprecedented pace, with the global artificial intelligence market surpassing $184 billion in 2024, marking a $50 billion growth over the previous year. This rapid adoption, while promising, comes with significant risks that demand a dual focus on innovation and regulatory compliance.
Generative AI Governance is not just about adhering to regulations—it’s about ensuring ethical, fair, and secure use of technology that is reshaping industries. For instance, concerns about algorithmic bias, privacy breaches, and malicious misuse of AI outputs are not hypothetical but pressing realities. The global AI governance market reflects this urgency, projected to grow from $890.6 million in 2024 to over $5.7 billion by 2029 at a CAGR of 45.3%. This growth underscores the increasing demand for frameworks that mitigate risks while unlocking AI’s full potential.
In this blog, we will take a deep dive into the core principles of generative AI governance, explore governance models, and share best practices from V4C’s AI experts to help organizations implement effective and scalable solutions.

Why Generative AI Needs Governance
The Rise of Generative AI
Generative AI is revolutionizing industries with its ability to create content, synthesize data, and drive innovation. From large language models (LLMs) powering content creation to AI-driven advancements in drug discovery, the possibilities are vast. In healthcare, generative AI accelerates drug development by simulating molecular interactions, while in media, it automates personalized content generation at scale. These applications highlight the technology’s transformative potential but also underscore the critical need for governance to ensure its ethical and responsible deployment.
Key Risks of Generative AI
- Bias and Fairness Issues
Generative AI models can inadvertently perpetuate biases present in their training data. For example, biased lending algorithms have led to discriminatory practices in financial services, sparking concerns about fairness and equity in AI decision-making. - Data Security and Privacy Concerns
As generative AI relies on vast datasets, it raises significant privacy challenges. The unauthorized use or exposure of sensitive information in training data could lead to breaches or violations of regulations such as the EU AI Act. - Risk of Misuse
Generative AI’s capabilities can be exploited for malicious purposes, such as creating deepfakes, spreading misinformation, or generating harmful outputs. These risks not only threaten public trust but also expose enterprises to reputational and legal liabilities.
Core Principles of Generative AI Governance
Transparency
Transparency is foundational to generative AI governance. Explainable AI tools, such as feature importance reports and pipeline lineage tracking, are essential for understanding how AI models make decisions. These tools empower stakeholders to identify and address biases, ensure fairness, and build trust with end users. For example, by maintaining a clear lineage of data and transformations, organizations can ensure the integrity of their AI systems while providing clarity to regulators and stakeholders.
Accountability
Structured accountability mechanisms, including sign-offs and approval processes, play a critical role in preventing irresponsible AI deployment. These mechanisms create checkpoints throughout the AI development lifecycle, ensuring that each phase—from model design to deployment—aligns with ethical guidelines and organizational objectives. By assigning clear ownership and responsibility, businesses can mitigate risks and respond proactively to potential failures.
Compliance
Compliance with regulatory standards, such as GDPR and the EU AI Act, ensures that generative AI operates within legal boundaries. Governance frameworks should include robust mechanisms for risk assessment, audit trails, and model documentation to meet these standards. For instance, the EU AI Act emphasizes transparency, risk management, and accountability, making it vital for organizations to adopt compliance-driven frameworks for AI development and deployment.
At V4C, we recognize the critical role governance plays in ensuring ethical and compliant AI solutions. Our experts work diligently to embed best-in-class governance practices into every AI initiative, ensuring adherence to regulatory standards and fostering trust in AI-driven innovation.
Governance Models for Generative AI
Centralized Governance Model
A centralized governance model ensures uniformity by consolidating policies and oversight under a single authority or team. This approach is particularly beneficial for large organizations operating across multiple regions, as it simplifies regulatory compliance and enforces consistent standards.
Example: Centralized registries for large language models (LLMs) can track model usage, monitor performance, and ensure compliance with organizational policies. This approach reduces redundancy and enhances control over AI initiatives.
Decentralized Governance Model
In a decentralized model, individual teams or departments take responsibility for their AI initiatives. This approach offers greater flexibility and agility, making it suitable for smaller organizations or teams working in fast-paced environments.
- Pros: Encourages innovation and allows for tailored solutions that meet specific needs.
- Cons: Can lead to inconsistent practices and challenges in achieving compliance at scale.
Hybrid Governance Model
A hybrid model blends the strengths of centralized oversight with the flexibility of decentralized governance. Centralized bodies establish core guidelines and policies, while local teams retain autonomy to innovate within these boundaries.
Example: Platforms like Dataiku enable hybrid governance by providing centralized project tracking and monitoring tools while empowering local teams with the autonomy to experiment and innovate. This model strikes a balance between consistency and agility, ensuring that generative AI initiatives are both compliant and innovative.
Recommended Read: Real-World Generative AI Applications Across Industries: With Actionable Insights
Best Practices for Generative AI Governance
Implementing effective governance practices for generative AI ensures ethical, reliable, and compliant AI operations. Here are some best practices to consider:
- Centralized Oversight with Tools Like Dataiku Govern: Use platforms such as Dataiku Govern to centralize project tracking and model oversight. These tools provide visibility into AI workflows, ensuring alignment with governance standards and simplifying compliance.
- Bias Detection and Drift Analysis: Regularly analyze models for bias and drift to maintain fairness and reliability. Continuous monitoring helps identify unintended consequences and enables corrective measures before deployment.
- Explainability Tools: Leverage explainability features, such as model interpretability reports and visualizations, to foster trust among stakeholders. Transparent AI systems build confidence and encourage stakeholder buy-in.
- Value and Risk Qualification Matrix: Define a matrix to evaluate the potential value and associated risks of AI projects. This prioritization tool ensures resources are allocated to initiatives that align with organizational goals and compliance requirements.
V4C’s Expertise in Generative AI Governance
V4C is a trusted partner for organizations aiming to implement scalable and effective governance frameworks for generative AI. Leveraging platforms like Dataiku, V4C ensures businesses can innovate responsibly while maintaining compliance.
How V4C Supports Generative AI Governance
- Framework Development: V4C designs comprehensive AI governance frameworks tailored to your organization’s regulatory and operational needs.
- Tool Implementation and Optimization: From setting up platforms like Dataiku Govern to optimizing workflows, V4C equips businesses with the tools and strategies needed for effective oversight.
- Continuous Monitoring and Enablement: V4C provides ongoing support, including monitoring models for compliance, enabling rapid adaptation to new regulations, and ensuring long-term governance readiness.
Conclusion
The rapid adoption of generative AI offers unparalleled opportunities for innovation across industries, but it also presents significant governance challenges. As organizations strive to balance the demands of creativity and compliance, a robust governance framework becomes essential to mitigate risks, ensure fairness, and maintain trust. From establishing transparency and accountability to adhering to global regulatory standards, effective governance is the foundation for responsible AI development and deployment.
Partner with V4C.ai today to implement robust generative AI governance frameworks and ensure your AI initiatives are transparent, accountable, and compliant—driving innovation responsibly and effectively.
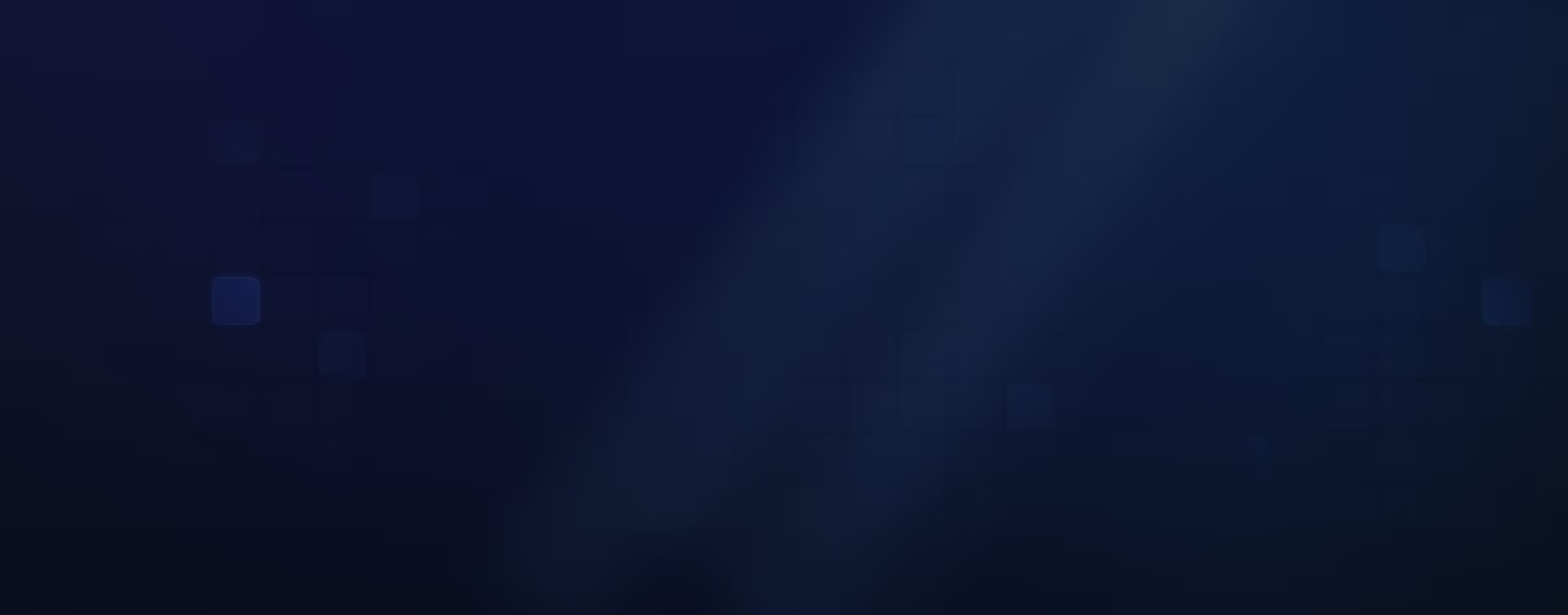